This work addresses the challenges in the practical application of the Quantum Approximate Optimization Algorithm (QAOA) due to current limitations on quantum computational resources. The authors propose optimizing QAOA initialization using Graph Neural Networks (GNN) as a warm-start technique, sacrificing affordable computational resources on classical computers to reduce quantum computational resource overhead and enhance QAOA’s effectiveness. Experiments with various GNN architectures demonstrate the adaptability and stability of the proposed framework, highlighting the synergy between quantum algorithms and machine learning. The findings show GNN’s potential in improving QAOA performance, opening new avenues for hybrid quantum-classical approaches in quantum computing and contributing to practical applications in solving complex combinatorial optimization problems, such as the Max-Cut problem. PDF
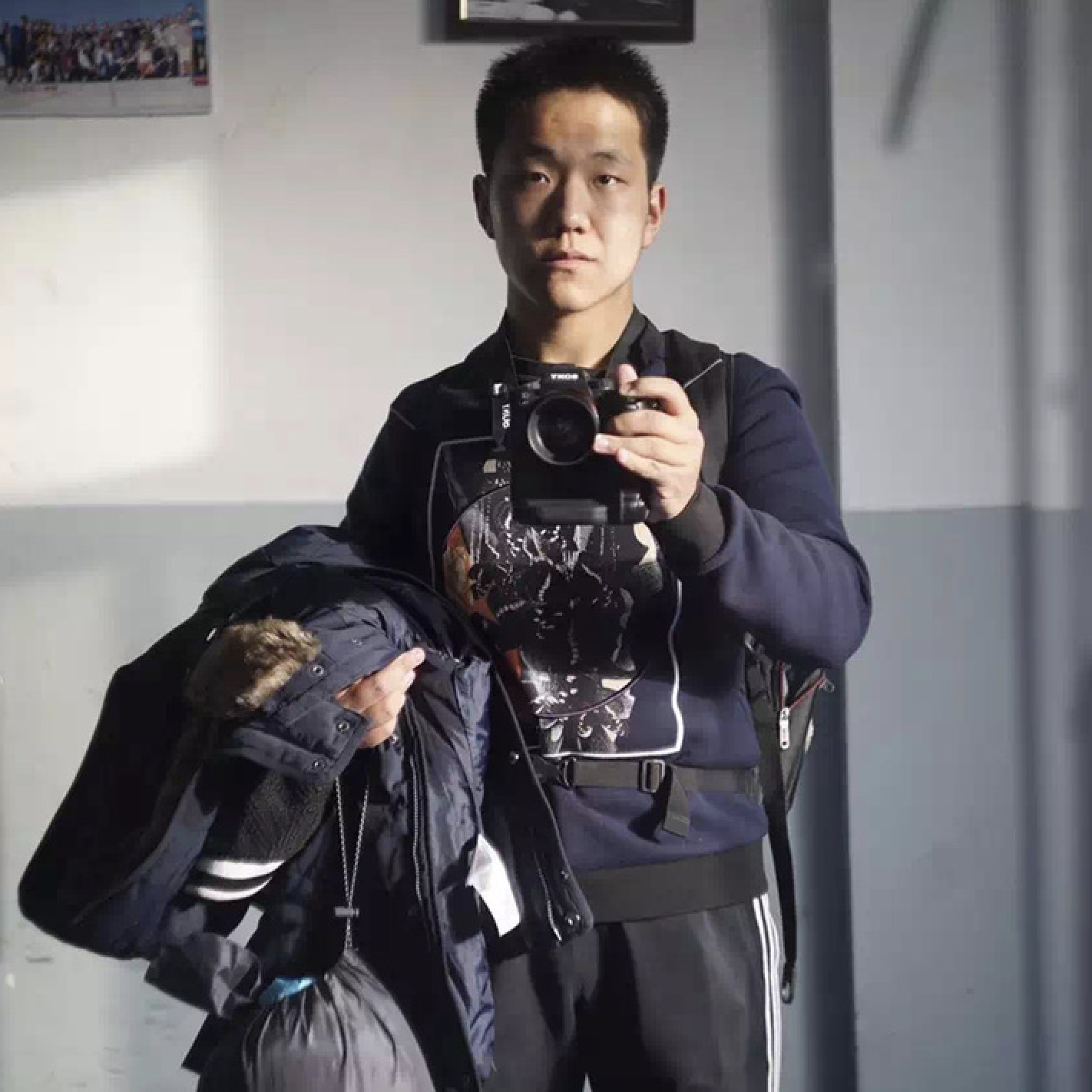
Jinglei Cheng (J.C.)
I'm a CS PhD student at Purdue University. My research interests include quantum computing and computer architecture.
- West Lafayette, IN, United States
- ResearchGate
- Github
- Google Scholar